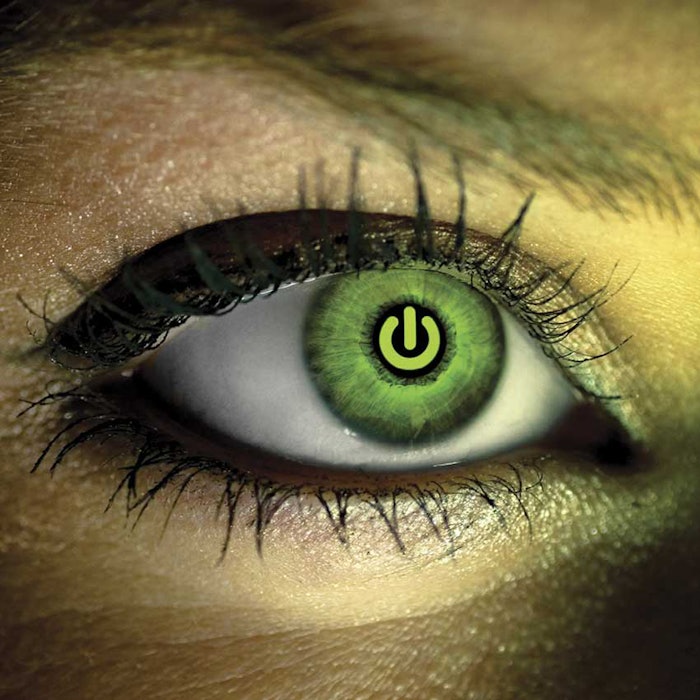
Big Data is everywhere, tracking click-throughs, web cookies and time stamps of our daily lives like the digital paparazzi. It can be used for good, revealing patterns in the most miniscule of details and leading to discoveries beyond our wildest imaginations. But in the wrong hands, it’s dangerous; unraveling identities, draining bank accounts or worse.