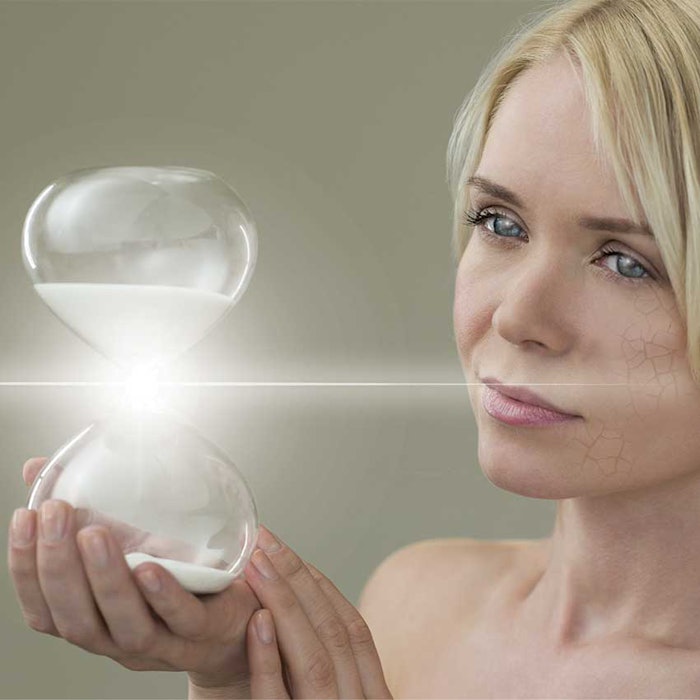
The age distribution of the world’s population is dramatically shifting; longevity rises while fertility rates remain flat. According to demographic studies, 2020 will be a turning point, wherein individuals 60 years and older will outnumber children younger than five. Today, it is no longer a rarity to live 80 years or more in many parts of the world.1
This increasing longevity has led to new challenges in the medical field. Today’s population expects to live longer and in good health—i.e., without facing the declines previously associated with aging. This status is referred to as healthy aging, aging gracefully or aging well;2 it was defined by Rowe and Kahn as freedom from disease, high cognitive and physical functioning, and a rich social life.3
An added element of aging well is one’s perceived age. In elderly individuals, a perceived age lower than their chronological age is associated with high survival.4 Among consumers 50 years and older, there are two main outlooks on the signs of aging. The first is negative, where individuals do not accept their age and seek to erase the signs of time; e.g., the use of anti-aging products, in attempt to look younger.5 The second is positive, where individuals assume aging as part of their personal identity. The latter embraces the beauty of maturity; for example, by using skin care that harmonizes their perceived and chronological ages.6 From this trend, a new dermo-cosmetic concept emerged, well aging, which focuses on wellness in order to maintain health capital.
Although aging affects all organs, judgements that estimate age are primarily—and more easily—based on different facial features. These play an important role in human identity and social interactions. For example, evidence exists that visible facial skin conditions influence the perception of age and health as well as attractiveness. Interestingly, regardless of race, nationality or age cues, humans are highly sensitive to skin color distribution and surface topography; variations in these features occur due to intrinsic and extrinsic aging processes.7, 8
For instance, within Caucasian populations, a youthful face exhibits consistent textures and smooth facial contours that merge together harmoniously; sagging skin and the appearance of wrinkles and pigmented spots are the most visible changes associated with aging.9, 10 Indeed, the two major fibrous proteins of the skin deteriorate with aging, resulting in the loss of elasticity and the formation of fine lines and wrinkles, particularly in areas subjected to continuous or repeated tensions. Moreover, an increase or decrease of melanocytes in specific areas due to oxidative stress causes visible heterogeneity in melanin distribution, leading to the formation of pigmented spots.11
Despite having successfully identified the signs of aging, there is no concrete evidence to explain why some individuals look older than their chronological age and others look younger. As such, it would be interesting to determine which cutaneous characteristics contribute to the perception of age. A few studies have focused on the role of age-related skin features in appearance, but these have only considered lifestyle and environment.12 Another study determined that eye, lip and skin color uniformity are important attributes to estimate a Caucasian woman’s age;13 however, no reports of in vivo biometric measurements have been described to differentiate those who do or do not age well.
In this context, the present study aimed to determine in vivo variations in cutaneous parameters that are associated with facial appearance and perceived age. For this purpose, researchers organized focus groups to collect definitions and expectations of consumers regarding the well-aging concept. Analyses of these discussions were used to create word clouds, from which artists used the four top descriptors to sketch a portrayal of individuals who had aged positively or negatively.
Once identified, these discriminating parameters were validated in vivo by instrumental measurements taken in a panel of 373 subjects. The results were plotted in a multidimensional scaling (MDS) matrix to generate a geometric representation of subjects according to their similarities or differences. By mapping in vivo variations in cutaneous parameters, subjects’ ages were then predicted and compared with chronological age. This enabled the identification of individuals demonstrating harmonized chronological and perceived age; i.e., well aging.
Methods: Focus Groups
Focus group: Focus groups were used to collect perceptions of well aging based on previously defined questions designed to stimulate different opinions.14 These were conducted between August and September 2016 and led by a neutral facilitator in the presence of an observer taking notes. Forty-two Caucasian women between the ages of 40–65 were divided into seven groups: two groups ages 40–45; three groups ages 45–55; and two groups ages 60–65.
Questionnaire: The well-aging concept was approached through short, open questions ranging from the most general to the most specific:
- If you had to name a personality whom you say has aged well, who would you choose?
- What criteria make you say they have aged well?
- If you had to name a personality whom you say has aged poorly, who would you choose?
- What criteria make you say they have aged poorly?
- Can you describe someone who has aged well?
- Can you give visual characteristics of someone who has aged well?
- According to you, how do you preserve skin so that it ages well?
- In your opinion, how can a cosmetic product help the skin to age well?
Qualitative analysis of the discussion: A retro-transcription of the immediate reactions was performed after the session by the facilitator and observer. The discussions also were recorded, transcribed and analyzed using softwarea. These approaches were used to uncover additional perspective content.
Materials and Methods: Biometric Assessments
Based on findings from the focus groups, biometric assessments were made in a consumer panel.
Panelists: Measurements were taken in 373 Caucasian women, ages 19–78 years. Analyses were performed on the face following at least 15 min of acclimation under control room conditions (22°C ± 2°C; 40% RH). Studies were conducted according to the Declaration of Helsinki Principles and were approved by an internal review board. All procedures for the recruitment, selection and inclusion of subjects were established to provide clear and precise information, allowing subjects to appreciate the aims of the project and consequences of their consent; informed consent was obtained from all participants.
For the same chronological age, a wide combination of cutaneous characteristics existed, suggesting the independent analysis of one criteria may not be relevant enough.
Crow’s feet: Silicone polymerb replicas were made of the subjects’ corner eye areas to obtain negative prints.15 This replica material contains a catalyst that allows it to condense into a strong, resistant and viscous material within 10 min. This silicon polymer pre-mix was applied to skin surfaces marked previously with a circular, double-sided sticker to indicate the exact site and orientation of the replica. The final replica was then covered by a clear, thin plastic foil and flattened on the back side into an even thickness.
Analyses of the replicas were performed by illuminating each at a 35-degree angle and capturing a photographc. At this angle, wrinkles become visible thanks to shadows created by the grazing light. Stages of wrinkling were then analyzed by a jury of experts, who reviewed the digital images and rated them.
A scoring scale of five stages (see Figure 1) was developed based on: microrelief, presence of fine lines, and depth and length of wrinkles. Stage 1 represented skin with no wrinkles and a uniform, regular microrelief, whereas Stage 5 represented large wrinkles and an irregular microrelief; the severity of the crow’s feet wrinkles therefore increased with increasing numbers. The overall score of the crow’s feet wrinkle stage corresponded to the mean of the scores attributed by each expert. Results of this semi-quantitative evaluation were expressed in arbitrary units (AUs).
Skin radiance: A blind test was performed by two experts previously trained to evaluate the following parameters on the cheekbones, forehead, chin and eyes: skin grain; transparency and reflection; red, olive and light pink color; state of eye fatigue; and presence of lines. The overall score for skin radiance corresponded to the global scoring of the entire face after individual evaluations of the parameters named. This was expressed on a continuous scale in AUs, ranging from 0 to 5; Stage 0 characterized a very dull complexion while Stage 5 characterized a very radiant complexion.
Biomechanical properties: Five successive cycles comprising 5 sec of suction and 5 sec of release were carried out on the face using a cutometerd with a 6-mm probe. The depth of penetration into skin was measured and transcribed by software. The obtained curves allowed for the calculation of different parameters such as total elongation (Uf) and residual deformation/total elongation (Ua/Uf), characterizing skin firmness and elasticity, respectively. A decrease in the Uf parameter characterized a tensing effect, indicating firmer skin, and an increase in the Ua/Uf parameter characterized an improvement in skin elasticity, which is associated with younger skin behavior. Results were expressed in millimeters (mm).
Cutaneous hydration analysis: The electrical capacitance of skin’s superficial layers also was assessed on the cheeks using a corneometere. Four measurements were taken per area at 5 sec intervals; results were expressed in AU.
MDS matrix: The results obtained for all four parameters—i.e., hydration, firmness, wrinkles and radiance—were translated into a distance or dissimilarity matrix, to score the difference between each pair of volunteers. These scores were projected into a 3D matrix through the mathematical method of multidimensional scaling (MDS), providing an optimized geometric representation of the similarities and differences between subjects.
In this representation, spatial coordinates are irrelevant since only the relative distance between each point is important.16 From the MDS representation, age regression was carried out according to positions in the MDS space, which allows for the representation of both age axes and calculates a predicted age for each volunteer.
Results: Well Aging, Defined
Mathematical analysis of the focus group discussions identified the 50 most-expressed words for each question. As an example, Figure 2 shows the word cloud obtained for the most frequently used words to describe someone who has aged well.
Analyzing the word cloud obtained for each question, aging well appears to involve notions of vitality and a natural way of life, according to these panelists. Secondary notions include charm, appearance and signs of aging.
More specific to cutaneous features, these panelists distinguished aging well or poorly according to four main traits: 1) hydration, 2) firmness, 3) the absence of wrinkles and 4) complexion radiance. Thus, these parameters were used to provide a sketch for whether an individual aged positively or negatively.
Results: Instrumental Measurements
As noted, the identified parameters were analyzed instrumentally. Data obtained for each volunteer regarding hydration levels, biomechanical properties, stages of crow’s feet wrinkles and radiance were collected (see Table 1). Results showed variations within these parameters between each volunteer, which surprisingly were not necessarily correlated with their chronological age. Thus, for the same chronological age, a wide combination of cutaneous characteristics existed. As a consequence, independent analyses of these four criteria may not be relevant enough, which suggests the evaluation of a subject’s whole profile could be more informative.
The panelists in this study expressed that aging well involves notions of vitality and a natural way of life, as well as charm, appearance and signs of aging.
Results: MDS Visualization of Well Aging
As previously described, volunteers were positioned in the MDS space in relation to each other and according to variations in parameters. Therefore, the position of each person corresponded to the dissimilarity of their results for the four cutaneous parameters (see Figure 3). Here, the MDS representation was further clarified by color-coding each volunteer according to chronological age.
Surprisingly, this figure shows that even without the ages of volunteers as discriminating criteria, the results were naturally arranged from the youngest, on the left side, to the oldest, on the right. This mathematical representation therefore highlights that the combination of hydration, firmness, wrinkles and complexion radiance provided an aging profile of subjects that was independent of their chronological age.
Results: Linear Regression for Age Prediction
Figure 3 also shows that some volunteers did not fall on the MDS matrix in an area more typical of their similarly chronologically aged peers; in fact, some older volunteers fell toward the center and even further to the left on the MDS. This suggested they had a younger appearance, so these individuals could be considered as having aged well. On the contrary, some whose chronological age (CA) was younger than their MDS placement, i.e., toward the center or right, could be considered to have aged poorly.
In order to confirm this hypothesis, based on selected cutaneous parameters, an age regression was carried out according to positioning in the MDS space. This allowed for the calculation of a predicted age (PA) for each volunteer; i.e.: PA - CA = Δ or the change in aging profile of volunteers. A negative result indicated the volunteer aged well whereas a positive result meant the volunteer aged poorly (see Table 2). From this analysis, 174 volunteers were perceived to have aged well and one volunteer’s appearance matched her chronological age. In conclusion, the results identified, for the first time, the in vivo variations of different cutaneous characteristics that contribute to a perception of well aging.
Discussion
A recent study demonstrated the negative impact that feeling older can have on health.6 Therefore, as the proportion of today’s population 80 years and older accelerates, it is important to determine the criteria involved in this negative outlook.2
The present study used a mathematical model to quantify features described by focus groups of Caucasian women14, 17 as affecting perceived aging. An in vivo sketch of these parameters was then developed and the results highlighted four areas discriminating well or poor aging: hydrated vs. dry skin; firmness vs. sagging; wrinkles (or not); and complexion radiance vs. dullness. Among these parameters, firmness, wrinkles and radiance had already been described in the literature.13 This validated the test panel as being representative of the Caucasian population.
Once validated, applying this model to biometric data could be a quick and inexpensive method to estimate perceived age while avoiding subjective human bias.
Surprisingly, the fourth parameter, skin hydration, is new to the well-aging concept since it is not a direct actor of perceived age. While the loss of hydration is a largely known consequence of aging, it has never been underlined by consumers as a factor in perceived age. Indeed, aging is linked to reduced cohesion in the stratum corneum and a decrease in proteoglycans such as hyaluronic acid. This leads to increased transepidermal water loss and further dehydration. Dry skin is also less elastic, which influences surface topography;18 although it should be noted that sensitization and consumer awareness regarding hydration could explain why it was used as a descriptor by panelists during the focus groups.19
These four characteristics—i.e., hydration, firmness, wrinkles and radiance—were then biometrically assessed in panelists and the results were considered as a whole, since individually, one could not accurately serve as the dominant feature describing perceived age. To combine these characteristics, the results were translated into a dissimilarities matrix, represented by MDS.16 This dimensionality reduction is crucial for analyzing and revealing the genuine structure hidden in the data.20
The MDS representation highlighted a natural arrangement of subjects according to age, even though this parameter was not used as input data. This confirmed that the four parameters identified from the focus groups were relevant to establishing the aging profile of subjects. Indeed, several publications have already addressed this issue but mainly as a function of lifestyle, such as sleep quality and quantity, smoking, body mass index and healthy diet; or environment, such as pollution and UV index.12, 21
The present study was carried out at the scale of biology and free from environmental influences. However, now that cutaneous parameters for perceived aging have been identified, it could be interesting to add these environmental components. Moreover, a recent study showed that women are remarkably sensitive to subtle variations in hair density, style and color—additional parameters that could be translated to the skin for further study.22
Taking the results even further, linear regression of the subjects according to their position in the 3D space allowed for the calculation the predicted age for each volunteer and discrimination between those who aged well vs. poorly, since they were respectively characterized by a negative or a positive change in perceived age; i.e., Δ = PA – CA. Further analyses are necessary to confirm that the age predicted by this mathematical model corresponds with what might be given by a third party. However, once validated, the application of this model to biometric data could be a quick and inexpensive method to estimate perceived age, while avoiding human subjective assignments exposed to individual bias.23
In the growing context of customized cosmetics, this approach could also be used to calculate the predicted age of each consumer in order to offer targeted products. Although since individuals of different ethnic origin do not use the same cues equally to describe perceived aging,24 identification of cutaneous features should be performed for each ethnic origin.
Conclusions
In conclusion, this work has identified a combination of four cutaneous parameters to describe well aging. This discovery will improve the ability to score perceived age and discriminate between aging well and poorly. The described model also could serve as a powerful tool to select relevant panels for the substantiation of innovative skin care products that achieve harmony between perceived and chronological age.25
Acknowledgements: The authors wish to thank all volunteers for discussions during the focus groups.
References
- W He, D Goodkind and P Kowal, An aging world: 2015, International Population Reports, U.S. Census Bureau (2016)
- M Gangbè and F Ducharme, Le "Bien vieillir": Concepts et modèles, Médecine Science 22 297-300 (2006)
- JW Rowe and RL Kahn, Successful aging, The Gerontologist 37(4) 433–440 (1997)
- I Rippon and A Steptoe, Feeling old vs. being old: Associations between self-perceived age and mortality, JAMA Internal Medicine 175(2) 307–309 (2015)
- Get old—Dans vieillir, il y a Vie, press release, Pfizer (2014)
- NF Woods, E Rillamas-Sun et al, Aging well: Observations from the women’s health initiative study, J Gerontology series A, Biological Sciences and Medical Sciences 71 suppl 1S3–S12 (2016)
- A Nkengne and C Bertin, Aging and facial changes—Documenting clinical signs, part 1: Clinical changes of the aging face, Skinmed 10(5) 284–289 (2012)
- N Samson, B Fink and PJ Matts, Visible skin condition and perception of human facial appearance, Intl J Cos Sci 32(3) 167–184 (2010)
- MA Farage, KW Miller, P Elsner and HI Maibach, Intrinsic and extrinsic factors in skin aging: A review, Intl J Cos Sci 30(2) 87–95 (2008)
- AC Ko, BS Korn and DO Kikkawa, The aging face, Survey of Ophthalmology 62(2) 190-202 (2016)
- N Samson, B Fink and P Matts, Interaction of skin color distribution and skin surface topography cues in the perception of female facial age and health, J Cos Derm 10(1) 78–84 (2011)
- DA Gunn, H Rexbye et al, Why some women look young for their age, PLOS ONE 4(12) e8021 (2009)
- A Nkengne, C Bertin et al, Influence of facial skin attributes on the perceived age of Caucasian women, JEADV 22(8) 982–991 (2008)
- J Sharken Simon, How to conduct a focus group, The Grantsmanship Center Magazine (9)1–10 (2003)
- DH Kim, YS Rhyu, HH Ahn, E Hwang and CS Uhm, Skin microrelief profiles as a cutaneous aging index, Microscopy 65(5) 407–414 (2016)
- MC Hout, MH Papesh and SD Goldinger, Multidimensional scaling, Wiley Interdisciplinary Reviews, Cognitive Science 4(1) 93–103 (2013)
- R Merton, M Fiske and P Kendall, The focused interview. A manual of problems and procedures, The Free Press, Glencoe—Bij tij en ontij (1956)
- U Blume-Peytavi, J Kottner et al, Age-associated skin conditions and diseases: Current perspectives and future options, The Gerontologist, 56 suppl 2S230-242 (2016)
- www.mangerbouger.fr/Le-Mag/Bien-etre/L-eau-indispensable-a-notre-bonne-sante (Accessed Aug 17, 2018)
- J Tzeng, HH-S Lu and W-H Li, Multidimensional scaling for large genomic data sets, BMC Bioinformatics 9179 (2008)
- OH Franco, K Karnik, G Osborne, JM Ordovas, M Catt and F van der Ouderaa, Changing course in aging research: The healthy aging phenotype, Maturitas 63(1) 13–19 (2009)
- B Fink, C Hufschmidt, T Hirn, S Will, G McKelvey and J Lankhof, Age, health and attractiveness perception of virtual (rendered) human hair, Frontiers in Psychology 71893 (2016)
- M Coma, R Valls et al, Methods for diagnosing perceived age on the basis of an ensemble of phenotypic features, Clinical, Cosmetic and Inves Derm 7133–137 (2014)
- J de Rigal, I Des Mazis et al, The effect of age on skin color and color heterogeneity in four ethnic groups, Skin Research and Technology 16(2) 168–178 (2010)
- R Ganceviciene, AI Liakou, A Theodoridis, E Makrantonaki and CC Zouboulis, Skin anti-aging strategies, Dermato-Endocrinology 4(3) 308–319 (2012)